IS NECESSITY THE DRIVER OF INVENTION? NEED TO RESPOND TO NATURAL DISASTER AS SOURCE OF INVENTIVENESS IN THE HORN OF AFRICA.
Abstract:
Two conditions are essential for knowledge production in the Horn of Africa region, namely necessary condition (existing or new needs) and sufficient condition (capacity and ability). This paper attempts to investigate how and why necessity and capability have or have not created an opportunity for new knowledge or stock of knowledge to be created in the Horn of Africa region.
The paper also examines if necessity exists in the Horn of Africa’ There are recurring natural disasters in the region and there is expectation that there is a heightened motivation to adapt to natural disaster. Hence, the motivation to adapt can serve to spur invention and innovation. It is important to note that motivation to invent and innovate is not only limited to profit, as most economic models show. It could be driven by the desire to do social good and respond to natural disaster like earthquake, flooding, and drought in anticipation of future cost and discomfort avoidance.
preliminary findings show that R&D investment and human resource engaged in research boost invention and innovation in the region. However, due to serious problems with the data in the region, it is not possible to breakdown to specific countries or location.
In terms of knowledge spill over in the region, there is clear indication that the East African region (Kenya, Tanzania, and Uganda) exerts a significant influence on knowledge production in the Horn of Africa
With respect to the proxy measure of the impact of the disaster, like the number of people who died (death count) as well as number of people affected shows a negative, but insignificant influence on knowledge production as shown in all the Models. The situation is that unless supported by capacity and ability, necessity alone will not yield inventiveness in the HOA.
Introduction
The Horn of Africa region is prone to recurring natural disasters like drought and flooding. The four countries (Djibouti, Eritrea, Ethiopia, and Somalia) in the region have experienced one time or another drought and famine over the last 50 years. The most widely reported drought caused famines are the 1972-74 and 1983-85 famines in Ethiopia. During the 1972-74 drought, about 3,000,000 people were affected with about 100,000 deaths (EM-Data).
Similarly, close to eight (8) million (7,750,000) people were affected by the 1983-85 drought related famine with 300,000 deaths (EMA-data). Kumar systematically analyzed and shed light on the two epic famines of 1972-75 and 1983-85 in Ethiopia (Kumar, 1987). Table 1 and table 2 illustrates the drought and flooding experience of the Horn of Africa between 1964 and 2019.
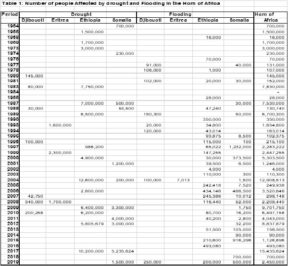
Source: The table is created form EMA- data
Necessity can be either basic ongoing regular needs for food, water, shelter, and good environment (clear air) or a continuously changing and evolving need due to external shock caused disruption to the basic needs, safety, and security. For instance, for communities in the Horn of Africa, drought and flooding is a frequent occurrence, and it is necessitating a constant and an innovative adaptation to the changing circumstances like need for food, shelter, and safety.
Under those circumstances, the creative thing to do is consider how to use the opportunities disasters create to deal with the current situation and prevent future disasters. One of the challenges to meet the needs in the HOA region continues to be the low level of skilled manpower in the region to learn from the disasters and convert the risks to create invention and innovation opportunities.
For instance, the Horn region experiences alternative cycle of flood and drought. Flood and draught are related. Under the right condition, resourcefulness and know how, it is possible to retain the flood water. The retained water through drainage technology and boreholes (wells) will create the possibility to deal with draught when the need arises. In effect, the flood becomes the solutions to counter the subsequent drought.
What compounds the skill shortage situation is that even after the region invest their meager resource to educate their population, due to lack of opportunity to utilize their acquired skills, a significant number of skilled people leave the region, resulting in a brain drain. For instance, Solomon have documented the brain drain from Ethiopia between 1970 and 1990 and demonstrated its impact on higher learning. (Solomon, 2002).
The hope is that those who left the region will one day return and bring their knowledge and skill to help the region invent and innovate. There is no clear indication that the return has happened yet. Brain drain is a big leakage to the region. A cursory examination of patent application in U.S and Europe shows several expatriates from Horn of Africa have been granted patents in areas like Pharmacy, exercise equipment, and Chemicals in North America and elsewhere.
Even if these expatriate from Horn of Africa are not returning, it is important to direct their efforts from wherever they are to tackle the acute needs of the region. As is demonstrated in other part of the world, natural disaster stimulates invention in the region by those who live outside of the region. We will investigate in another paper in the future what this leakage of skilled human capital means to Horn of Africa’s knowledge production function.
Overall, there is indication that innovation and patenting respond to natural disasters like flooding, drought, and earthquake. Natural disasters could incentivize those affected by the natural disaster to be creative to adapt to the disaster. Qing and Popp showed that adaptation to disaster stimulate inventiveness and patent flow to mitigate similar risks in the future. (Qing Miao David Popp, 2013).However, need alone may not lead to invention, “both motive and opportunity (need and knowledge) are necessary for invention to arise”. (Jacob Schmookler, (1962). Hence, in this paper regional economic knowledge production models are used together with motivation to adapt to disaster model to investigate inventiveness in the Horn of Africa region. Since the perception about the Horn of Africa around drought and famine is negative, it is a good to show there is more than hunger in the Horn.
Before we begin to build knowledge production function, let us examine how economics views knowledge. knowledge is mainly treated as a necessary and sufficient condition to reach and stay in equilibrium. If a new data becomes available that will alter the prevailing knowledge, then the equilibrium will not hold.
“If the tendency towards equilibrium, which we have reason to believe to exist on empirical grounds, is only towards an equilibrium relative to that knowledge which people will acquire in the course of their economic activity, and if any other change of knowledge must be regarded as a ” change in the data ” in the usual sense of the term, which falls outside the sphere of equilibrium analysis, this would mean that equilibrium analysis can really tell us nothing about the significance of such changes in knowledge, and would go far to account for the fact that pure analysis seems to have so extraordinarily little to say about institutions, such as the press, the purpose of which is to communicate knowledge”. (Hayek, F. A. von, 1937).
According to Hayek’s postulation of knowledge in economics. First, people acquire knowledge during economic activity (transaction knowledge). Second, data is the source of knowledge and if there is any change in knowledge, it must be considered as a change in data (link between data and knowledge). Third, there exists a division of knowledge (like division of labour) in such a way that no one person knows all (total knowledge is sum of individual knowledge). Fourth, knowledge acquisition is motivated by intention to carry out plan “in the course of the carrying out of his original plan” (knowledge is a plan execution tool). (Hayek, 1937).
Hence, further exploration revels that the economist’s conceptualization of knowledge and modeling is hence, constructed on the following guideline:
1. Knowledge is a Commodity. It satisfies the condition of being a commodity like tradability- bought and sold; individualized property (proprietary -patents), and re-producible, i.e. knowledge creates more knowledge.
2. In fact recent work has gone further to propose that due to not only its use value, but also for its exchange value, data (knowledge) could be considered as capital as well (Sadowski, Jathan, 2019)
3. Economic Development is a Knowledge (technological change) process: Even if early economic theories did not fully appreciate the role knowledge plays in economic development, more recent theories, especially dynamic theories are paying attention. For instance, in 1966, Boulding recognized the importance of knowledge in economic development as “It is only information and knowledge processes which in any sense get out from under the iron laws of conservation and decay,” (Boulding, 1966)
4. Decision (Economic system) Making is knowledge Driven: a decision is always a choice between alternative predicted outcomes of the future. The study of decision, therefore, must concentrate on how these outcomes of the future are derived from the information inputs of the past, as this is the only place from which they can come.
Since in general the models dealt with the production of knowledge with inputs, the specifications of the models varied depending on the inclusion or exclusion of input and output variables, the functional form, as well as the production technology’s assumption of return to scale. In this paper we will combine the regional inventiveness model and inventiveness as a response to natural disaster.
Literature review:
The literature review focus in three main areas. First, review the theoretical and empirical work around response to disaster and inventiveness, including economics of natural disaster, consequences, and responses to natural disaster. Second, review literature around regional invention and innovation in the HOA region, including motivation derived from adaptation to natural disaster. Finally, review the theoretical and empirical work that is done around invention and innovation in the drought and flooding prone Horn of Africa region.
The economics of natural disaster is an area that has gained significance recently. In terms of literature on the economics of natural disasters, their economic effects, and assessment of the determinants of natural disaster impacts, a good place to start is comprehensive review done by Miao and Popp (Miao, Popp, 2015)
More recently Botsen et al. reviewed the empirical studies concerning the economic impacts of natural disasters. They synthesized the main theoretical, computational, and empirical methods and findings, and discussed insights into factors and actions that have been found to mitigate disaster impacts. (Botzen, et al., 2019). However, there work is geographically limited.
Incentives in different forms play an important role in altering actions and reactions to extreme event. The incentive can take the form of forward-looking financial reward (profit) or reward by simply avoiding different types of future cost (cost avoidance). Both forms of rewards are determined after the fact, as no one for sure knows if the reward is realizable before it is actualized. In general, incentives are a function of the probability that is attached to the expectation and outcome.
Interestingly the power of incentive differs by type of disaster like earthquake, drought, and flooding. The incentivization also varies between local and distant locations. For instance, using U.S experience, a study examined risk avoidance and profit motive to explain innovation activities induced by natural disasters. It found out that drought and flooding damage “in a state does not necessarily spur innovations in local areas, rather, it stimulates impact-reducing innovations in more-distant locations.” (Hongxiu Li, 2018)
Besides incentives, converting disaster induced risk into meaningful action, like invention and innovation, requires appraisal of risk perception, response capacity and mechanism. A meta-analysis that was done recently questions the simple direct effects model of risk appraisal, and suggest “behavior change interventions will be most effective when they exploit synergies within the elements of risk appraisal, and between risk appraisals and variables such as coping appraisals” (Sheeran, Harris, Epton, 2013)
One way to address the coping appraisals is to gain knowledge. While disaster risk heightens the willingness to implement adaptive measures, availability of knowledge can solidify the required action, be it invention and or innovation. While reviewing the literature around knowledge production function, Griliches underscores pioneering knowledge source as “the publication of The Rate and Direction of Inventive Activity (Nelson 1962), a volume that still serves as a major statement and source book of economic ideas in this field.” (Griliches, Zvi, ed., 1984) (emphasis added)
Following the publication of the rate and direction of inventive activity in the early 1960s, Griliches laid his own foundational work by examining patent production at the firm level. (Griliches, 1979). Since Griliches work, others expanded and extended his work around knowledge production function (Romer, 1990, Porter and Stern, 2000).
What needs to be added in the knowledge production function besides R&D, and Human capital, spill over and externalities is the impact of external shock like adaptation to natural disaster. Often external shocks are captured in the unobservable variables. The challenge with that conceptualization is the external shocks like natural disaster are not uniformly distributed and cannot be assumed away as unobservable or externalities in places like the Horn of Africa. Hence, we will develop the framework to explicitly incorporate natural disaster risk mitigation in our conceptual and empirical analysis.
The final focus area for our literature review is the work done in the Horn of Africa region with respect to regionally focused knowledge production function with risk mitigation adaptation consideration. the regional-level studies based on the KPF is a mostly concentrated around North America and European regions, with few works sprinkled for Japan, China, and Korea.
As to the horn of Africa, even if not directly around country specific or the Horn of Africa region based knowledge production function, some work have been done around questioning conceptualization of natural disasters and food security (Sandstrom, Juhola, 2016) and Natural Disaster’s mitigating impact, Managing Risks in seven sample countries including Kenya (Laframboise, Loko, 2012), as well as local innovation studies in Kenya ( Boithi, et al, 2014).
One main challenge for empirical work in the region is chronic shortage of data. Recently a promising work to deal with data problem is emerging, such as the empirical method for selecting relevant attributes for modeling drought. This data was developed from experimental analysis using real world drought data for Greater Horn of Africa (GHA) (Getachew, Tsegaye, and Yared, 2017) This is an encouraging development that could hopefully stimulate more empirical work in the Horn of Africa region to bridge the chronic data gap.
Conceptual Framework
The Horn of Africa is an old and traditional region where countries in the region have their own alphabet and number system. The region is also prone to major natural disasters like drought, flood, and war. Communities in the region consider disaster as the “last resort after advice fails to force people to change their behaviour”. The traditional saying ‘advice someone once, twice, and three times, if they listen and change well and good, if not let disaster and hardship teach them’. There is expectation that disaster could eventually alter pattern of behavior to attain better outcome. This may include inventing and innovation out of natural disaster induced hardship.
Learning and adapting from Disaster has been part of the culture of experience-derived learning. The question is how is the knowledge gained from the disaster experience converted into invention and innovation? There are different theoretical propositions as to how under normal circumstances knowledge is produced at the regional level. It would be interesting to examine how the process works under disaster induced creation environment.
There are primary and supplementary factors that affect knowledge production at a regional level. The primary factors are the inputs like R&D expenditure and Human Capital that is devoted to R&D (Porter and Stern, 2000, Hart, Rob, 2016). Supplementary factors include: concentration of skilled workers in one place (Mellander, Florida, 2012); higher density of economic exchanges and contacts -agglomeration (Carlino and Kerr, 2014); institutions and strategies for technological changes (FUENTELSAZ, GARRIDO, MAICAS, 2014); and knowledge stock available in the whole economy as well as rest of the world and on the region’s ability to exploit it (Usai, Stefano, 2008)
Besides the conducive conditions for knowledge production at the regional level, there are also unique opportunities such as natural disasters that could prompt knowledge production to respond and adapt to calamities. Of course, the incentive for disaster prevention and damage mitigation measures including invention and innovation will depend on “the propensity with which a country experiences frequent and strong natural hazards.” (Neumayer, Plümper, Barthel, (2014))
Hence, conceptually it is important to bring together the conducive conditions fostered regional knowledge production function with unique opportunities that arises due to a need to mitigate disaster risk as a joint production function. This move of recognizing and aligning knowledge production with disaster risk mitigation is consistent with emerging areas of knowledge coproduction approach (Reyers et. Al., 2015) and Joint Knowledge Production (JKP) proposed to reconcile the supply and demand for knowledge (Hagger, D., C. Dieperink, 2014).
Empirical Estimation Modeling
The aim is to empirically test the hypothesis that risk mitigation can influence knowledge production in the Horn of Africa region on top of inputs like R&D expenditures, Human Capital, and stock of regional and rest of the world knowledge. We first start by specifying a general form of Horn of Africa region knowledge production function. This will provide us with the estimation of the production function without the risk mitigation factors. A reduced form of equation can be estimated as expressed in Charlot et. al. (Charlot et al, 2014)
I_t=ψ(RD_t,HK_(t,) WRD_(h,t,) WHK_(h,t,) U_(h,t)) (1)
where I_t is regional patent intensity in time t, ψ is a real function (often assumed to be a Cobb–Douglas function), h is 1; . . . ;N is an index of the neighbouring regions, t is 1; . . . ; T indicates time, RD_(h,t),HK_(h,t,) are R&D spending and HK at the regional level, are WRD_(h,t,) and WHK_(h,t,)R&D and HK levels in the regional neighborhood, respectively, and U_(h,t) represents all unobservable factors that influence regional innovative performance.
This equation has its roots from the framework of firm level production function that was initially proposed by Griliches, (Griliches, 1979). When it comes to the mechanics of the equation at the Horn of Africa region level, there are additional factors that can be introduced such as knowledge spill overs from neighbouring regions (East Africa, North Africa and African region), externalities like markets for invention and innovation, and economies of scale due to concentration (agglomeration). While it is adequate for our purpose to stay with general basic model, one factor that is important to include is the stock of knowledge in the region and outside of the region.
〖 I〗_t=ψ(RD_(,t),HK_(t,) WRD_(h,t,) WHK_(h,t,) 〖,SK_(t-1),SK_(n,t-1),U〗_(h,t,)) (2)
SK_(t-1) and SK_(n,t-1), are stocks of knowledge in the Horn of Africa region and outside of the region, respectively. The next step is to estimate the risk mitigation model. Following Maio and Popp, specify estimation equation for perceived risk as follows:
R*t =f〖{H〗_t C_t D_t 〖(M〗_t C_t)} (3)
Where 〖R^*〗_t is the perceived risk which is unobserved, H_t is Horn of Africa region’s baseline hazard (e.g. does the region have a fault line?), Ct is the region’s capacity to cope with a disaster in year t, and t Dt is the impact of current events (measured by death tolls or economic damages). The disaster impact D_(t )in turn depends simultaneously on the magnitude of the exogenous disaster shock Mt and the regions’ adaptive capacity Ct. (Miao and Popp, 2014).
Before proceeding to estimate the joint risk mitigation and regional knowledge production function, following Miao and Popp, let us estimate the adaptive capacity the region has.
C*t = f (H, Kt,Y_t,I_t) (4)
Where C*t is adaptive capacity of the region in time t, H is the baseline hazard, Y_t is income of the region, I_t is the region’s institutional responsiveness and ability, and Kt is the current knowledge and technologies available to cope with the disaster in question. If the current technology is unable to cop with the risk, then that may lead to invention and innovation.
Then by substituting equation 4 in 3 minus the Hazard baseline, H, to avoid double dipping as it is already included in equation 4, the perceived risk estimation equation with adaptive capacity built in as follows:
R*t =f{C_t^* D_t 〖(M〗_t C_t^*)} (5)
The final step to arrive at the regional knowledge production function estimation equation is to join the perceived risk equation 5 and the regional knowledge production function equation 2.
〖 I〗_(h,t)=g(RD_t,HK_(t,) WRD_(h,t,) WHK_(h,t,) 〖,SK_(t-1),SK_(n,t-1),R_t^*,U〗_(h,t,)) (6)
We will test out different functional form like additive, multiplicative functions with different returns to scale., One consideration is that due to very low levels of regional R&D expenditure, the patent and the R&D relationship could be flat, suggesting a low threshold to produce innovation.
Another consideration to keep in mind is that modeling the complexity of the invention and innovation process, especially in the Horn of Africa region, is a relevant challenge for this type of empirical analyses of innovation and its geography. Particularly due to lack of data, carrying out quantitative analysis is a daunting task.
In term of the heterogeneity of unobserved time-varying regional conditions linked to institutional quality, and organizational features, we hope that it is captured by the perceived risk mitigation specification. We will test out if this is born out by our estimation.
To our knowledge, Horn of Africa’s regional position in knowledge networks and nonspatial proximities to other innovative regions is weak. In the final specification we make sure that the empirical model can also account for the nonrandom selection of location decisions of innovative agents.
Countries within the Horn of Africa region are likely to differ with respect to some unobservable time-varying country-specific variables that are related both to the production of patents and to R&D and HK. We will try to account for that as well.
Datasets and Estimation
The analysis covers the four countries in the Horn of Africa region, namely Djibouti, Eritrea, Ethiopia, and Somalia. Ethiopia is by far the larger of the four countries while Djibouti is the smallest in the region. Eritrea was part of Ethiopia until it gained its independence in 1993. Besides geographical proximity, one common characteristic all four countries share is that they are prone to natural disaster like recurring drought, flooding, disease, and insect infestation(locust).
Except for Djibouti that relies heavily on port services, all the other three countries heavily rely on rain feed agriculture and pastoral herding. In terms of the size of the population and economy, Ethiopia is by far the biggest of the four. They all are at different stages of diversifying their economies. Most of the countries are embarking on building the resource extraction (potash mining in Eritrea) and building industrial parks (Ethiopia) and offshore oil drilling (Somalia) to promote foreign direct investment.
One of the biggest challenges to conduct empirical study in the Horn of Africa region is the inadequacy, reliability, and accessibility of data. Not only are there no consistently collected data, but also those that are collected are kept in silos and difficult to access, extract and compile them. For this paper, attempts were made to obtain data from credible sources.
When there are missing data, we tried to back cast and forecast to fill the missing values. This may introduce some bias to the data; however, we consider this to be a better option rather than a missing value and reduced number of observations.
The indicators for invention and innovation activity have been identified as the number of Patent Cooperation Treaty (PCT) patents and Number of Utility Model (UM) application and patents granted. The PCT and the UM patent we used are the one that are consistently available and internationally comparable patent data downloaded from the Intellectual Property Organization (IPO) database. The regional patent data is the sum of the four countries patents, where patents are available for each country and when it is available.
PCT and UM patent applications, grants and patents in force are available for 16 years from 2000 to 2016. As the dependent variable we used the patent intensity which is the number of patent application expressed per population of the region.
Disaster impact is measured using both number of people affected and human mortalities due to the natural disasters. Disaster data on the two types of natural disasters (drought and flood) is taken from EMA-data. Hence, the drought and flooding data is sourced from the Emergency Event Database (EMA-DAT) that is maintained by the Centre for Research on the Epidemiology of Disaster.
Unfortunately, since these are based on incident reporting mechanisms, questions have been raised about the quality of this data. As to the explanatory variables like R&D spending, Full time Equivalent employees working in R& D (Human Capital), The data is not reported consistently in the HOA.
Hence, using different estimation models, tested different options like using the value that is reported, replacing the reported value with a dummy variable corresponding to the time the R&D and human capital was reported. We also used spending on R&D and human capital (FTE) in Agriculture to capture the over all R&D and Human capital investments as a percent of GDP. The Agricultural sector is the largest employer of R&D human capital in this region.
Finally, we included Gross National Income per capita to capture the economic opportunity to invent and innovate as well as disaster risk mitigation capacity. The source of the R&D expenditure, human capital and Gross National Income per capital data is from the Development Indicator of the World Bank.
See table 3 for summary of the dependent and independent variables and their coverage and sources of data.
Table 3: Dependent and Independent variables For Estimation